While the study achieves remarkable prediction accuracy, the dataset’s size (18 experimental samples) raises concerns about overfitting and the generalizability of the hybrid model. Could the authors clarify how they validated the robustness of their model against such a small dataset? Furthermore, Figure 14 highlights improved prediction accuracy of the hybrid model, but there is limited discussion about potential biases introduced by WGAN-generated samples. How was the similarity between generated and real data assessed to ensure meaningful augmentation?
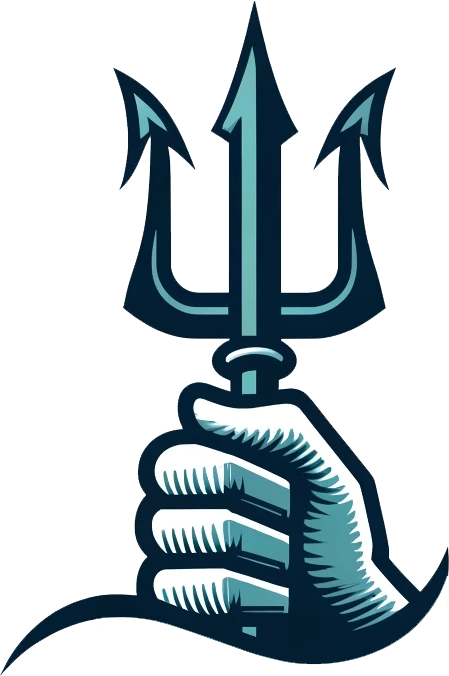
That’s a really valid concern! With only 18 experimental samples, it’s fair to question whether the model might be overfitting or if its predictions would hold up with a larger dataset. I assume the authors used cross-validation or some other technique to check robustness, but it would be great to get more details on how they handled this limitation.
Also, on the WGAN-generated data, Figure 14 shows strong accuracy improvements, but it’s not clear how well the synthetic samples actually match the real ones. Were similarity metrics, like distribution comparisons or feature space evaluations, used to confirm that the generated data truly enhances model training rather than introducing biases? Clarifying these points would help solidify confidence in the hybrid model’s reliability.