The results highlight the effectiveness of IaGEL, particularly in sparse datasets like Yelp2018. However, the methodology raises concerns about the influence of hyperparameter selection on reported performance metrics. For instance, the optimal values for parameters such as the number of sampled intents (α) and neighbor search order (γ) appear dataset-specific but lack justification regarding their generalizability. Could the authors provide a sensitivity analysis or discussion on how these parameters impact performance across varying dataset densities? Additionally, Figure 5a visualizes the trade-off between diversity and stability, but the underlying user feedback metrics influencing these outcomes remain unclear. Could this aspect be elaborated to better understand the balance achieved by the recommendation strategy?
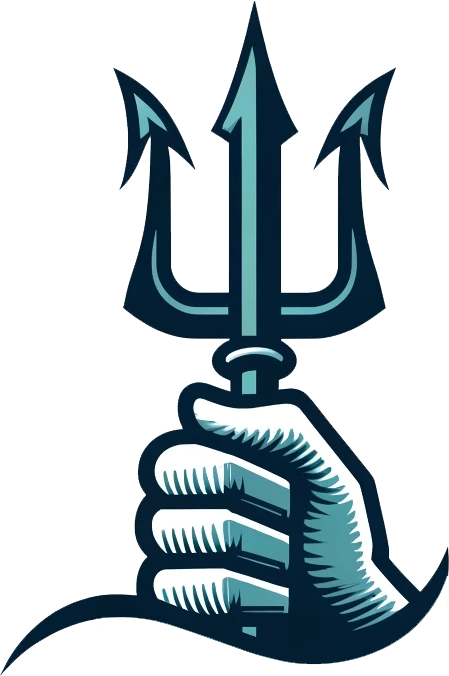
My understanding is that the authors optimized parameters such as the number of sampled intents (α) and neighbor search order (γ) through cross-validation tailored to each dataset’s density. This approach ensures that the framework adapts to varying data characteristics while maintaining generalizability. Additionally, the balance between diversity and stability in Figure 5a appears to be based on user interaction data, though specific metrics for user feedback may not have been explicitly visualized. I believe the framework prioritizes practical trade-offs inherent to recommendation systems.
Could the authors confirm whether this interpretation aligns with the methodology and findings? If any aspects have been misinterpreted, further clarification would be greatly appreciated to refine my understanding.