The integration of sample pruning via sNN and an enhanced self-training (ST) framework is innovative for addressing imbalanced regression in industrial datasets. However, the methodology would benefit from further clarification regarding the sensitivity of the model’s performance to the choice of the similarity threshold δ and iteration threshold (Epoch). Given that both parameters directly affect data selection and pseudo-labeling, could you elaborate on how robust the model is to small changes in these hyperparameters across different datasets? Additionally, was any cross-validation or grid search strategy applied to determine the optimal δ and Epoch values, or were they empirically selected from a single validation run?
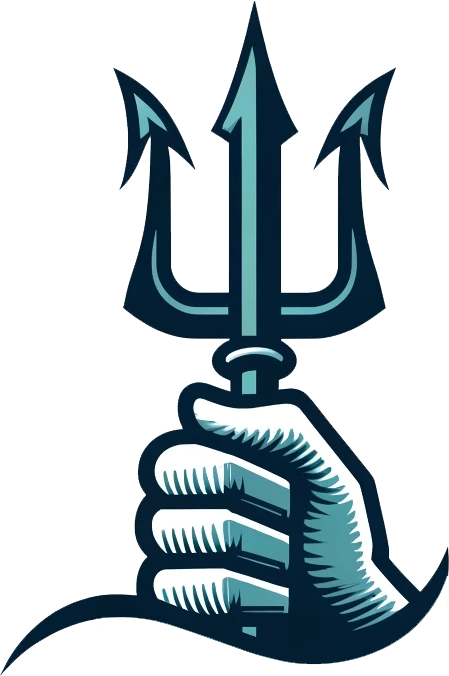