The paper lacks clarity in its AI training and validation processes, making reproducibility challenging. Some claims on AI applications in egg morphology lack strong empirical support, making conclusions appear speculative. The absence of discussion on potential biases raises concerns about real-world applicability. Strengthening methodological rigor and validation would improve clarity, transparency, and reliability.
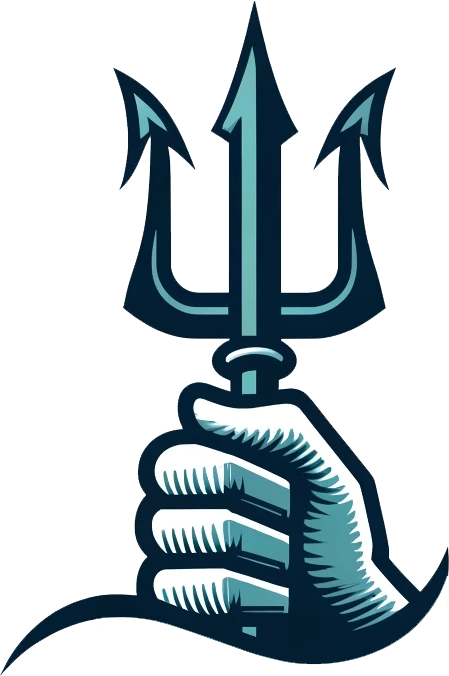
The concerns about AI training and validation are valid, as the paper lacks detail on dataset size, preprocessing, and performance evaluation. More transparency here would aid reproducibility. The study presents interesting AI applications in egg morphology, but stronger empirical validation, like comparisons with traditional methods, would bolster the conclusions. Discussing potential biases in AI training, such as dataset representativeness, would also enhance real-world applicability. Could the authors clarify how the AI model was trained and validated, including dataset size, augmentation techniques, and performance metrics used?