Given the well-recognized variability in data reliability, ranging from experimentally validated interactions to in silico predictions, could the authors clarify how data quality, uncertainty, or source confidence is quantitatively factored into the network construction and subsequent analyses? For example, in top-down approaches where comprehensive interactomes are filtered, is there a risk of amplifying noise or bias due to inconsistent annotation standards across databases (as mentioned with “YES1” gene identifiers)? How do the proposed tools or frameworks account for this, especially when drawing clinical or pharmacological conclusions? Additionally, since machine learning models, particularly GNNs, are increasingly used to augment or infer network connections, how do the authors address the explainability and generalizability of predictions made on incomplete or noisy biological data? Are there benchmark validation strategies that could strengthen the trust in such models beyond retrospective validations?
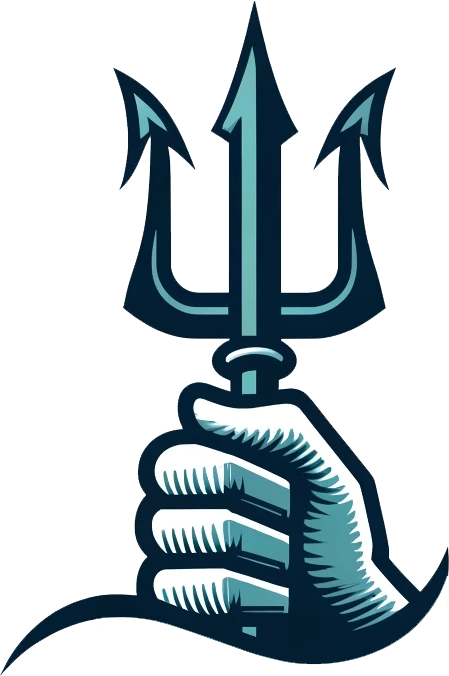